Welcome to No Jitter's ongoing series, Conversations in Collaboration, in which we speak with executives and thought leaders about the key trends across the full stack of enterprise communications technologies. Interviews in the series so far include:
- Cisco's Lorrissa Horton, , who talked about the company's approach to data security in AI.
- Google Cloud’s Behshad Behzadi, who spoke about the use of generative AI (Gen AI) at Google in its Contact Center AI offering and, more broadly, across enterprises.
- Amazon Connect’s Pasquale DeMaio, who discussed Connect’s history of AI usage and how it’s used in their contact center offering. Then came
- Calabrio’s Joel Martins, who talked about current AI implementations in auto-scoring and scheduling and provided a glimpse of how generative AI can help contact center managers, and
- IntelePeer’s Frank Fawzi who discussed how current AI implementations can be used to triage customer interactions before they reach the contact center and how generative AI can further improve that process.
(Editor's Note: Artificial intelligence has a lot of specific, descriptive terms. Download our handy guide to AI vocabulary here.)
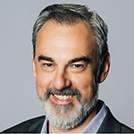 |
For this Conversation, NJ spoke with Brett Weigl, Senior Vice President and General Manager, Digital, AI and Journey Analytics at Genesys. Weigl oversees the company’s digital-first solutions for complete customer experience and AI across both digital and contact centers. Previously, he led the Digital Engagement product management team at Salesforce Service Cloud. |
No Jitter (NJ): Can we start with a brief synopsis of where Genesys sits, how you've been using AI in the contact center and your offering in general?
Brett Weigl: Everything we're talking about here is Genesys Cloud CX, which is our native built, born-in-the-cloud platform. It's been around since about 2016/17 and now has about 5,000 customers on it, many of whom use at least one AI feature.
As we build, we have two approaches generally. One is the ability to use native feature sets – we have a microservices architecture, so we build services around models that are appropriate for the use case that we are developing for. We also have a “bring your own set of options” available and we have an active ecosystem with many ISVs in it.
So sometimes, you know, you'll have an area like knowledge where someone will bring something specific that is maybe it's vertically optimized, or it has certain language support characteristics, or it’s popular in a particular geography. There’s a mutual advantage to Genesys integrating with those vendors. Third party bots are an example. Those are very common, although [the adoption] of our native chatbot and voicebot has now exceeded the adoption of the third parties.
But there is that mix and match, right? We have some clients who come and say, “Hey, I have a bot. It's really successful. I don't want to rebuild it. I just want to integrate it.” And they can do that. If you're at the point where you want to reboot your approach or you don't have anything, then you use [our] native [solution] – and that's increasingly the trend.
So, yeah, our AI portfolio has existed for several years. It has predictive elements that we use for predicting paths for customer engagements, effectively targeting and finding an intent and then directing to the best resource. We have predictive routing, which is very similar but once we understand the intent, it gets [customers] to the right agents. Those are machine learning kind of capabilities, not generative AI. It's just machine learning in the pure mathematical and outcome scoring sense.
We also have conversational AI, which is really three things. There's the ability to do voice bots and chatbots – [that’s more the] self-service approach. The [second is] agent assistive technologies, [which includes] an understanding [of] what is being talked about. [The third is] the conversational intelligence that you can glean from listening to the audio, but also reading through all of the transcripts of everything that's going on. So that's in general what the AI suite does today.
We've used the precursors to today's latest generative AI models since about 2020. So these are earlier models from Facebook and Google, BERT and RoBERTa. In the generative space, you have a concept of a foundational model, so there's a company that actually trains the underlying model. Then as a vendor, and this is what we've done, take that model and run it on our own platform, and we have a way to tune it.
This is especially true of anything from an open source ecosystem. So you take, effectively, a copy of the foundational model, tune it against your own corpus, and then you have additional options for customers to be able to further personalize what you've done.
NJ: How does that fine-tuning of the foundation model work?
Weigl: You have a foundational model that goes through a set of training – look at what OpenAI have been doing as an example. GPT 4 had some months of training on a huge amount of content and about $10 billion of compute on Azure to get it to that point. Once that training process is done it's not trivial to redo it, right? So, they filed the patent for GPT 5 and it'll take another, who knows, two or three months to train the next version.
The refinement really comes in where I contract with them and use whatever their latest foundational model is, but now I can run my own services on the side that effectively refine what its inputs and outputs are. [My services] effectively pre-prompt and they train it on my service characteristics or the applications that I am likely to build.
Say I have three or four use cases or, at a higher level, we could give the customer themselves the ability to tune. An example there would be what we do with “intents.” We allow them to take in their own work specific to their own tenant – which nobody else sees – and gives them the ability to extract from all of their transcripts the “meaning” in the form of intent and utterances.
Then they can go build a bot that [reflects] what their customers are actually asking for in all the phone conversations and digital conversations that they have powered by Genesys. That’s helped them to prime what their self-service experience is likely to be. Across our platform we've sprinkled [capabilities] that allow for you to generate the starting point with less steps and then also to learn from the results of trying to use that.
NJ: Can you provide a little more detail on the foundation models behind your generative AI efforts?
Weigl: We work pretty deeply with HuggingFace – basically they curate open source models. A lot of our latest features including the auto summarization for Agent Assist is based on a FLAN T-5 model and we're always upgrading the number of parameters [used] – the basic idea is that we're scoping in bigger and bigger improvements.
We also participate in Amazon Bedrock. Our platform is 100% written on AWS anyway, so we've already been using models, particularly on the machine learning side through SageMaker, but as you go into Bedrock they have a set of the top vendors – Anthropic, Cohere, etc.
What we’ve done – what we've always done – is use models that provide the best lift for the use cases we're tackling. Obviously within one feature, you don't want to hit you know, 80 different models. That's kind of a ridiculous [example], but we are quickly getting a sense of which ones work best in the contact center space.
In our sentiment analysis, and semantic search and topic mining, the way they work is they start with a generative model. But then we build tunings specifically for that use case and we run a service behind the scenes that's invisible to the customer but they get the feature – that’s really the takeaway.
NJ: Okay, so if an enterprise comes to you and says, I really want to use this particular large language model for whatever reason, is that something that you can enable?
Weigl: Not with our finished applications. With those, you’re using our out-of-the-box system. There are additional hooks we will build, though, so on our roadmap, we do have a “bring-your-own” generative AI model capabilities planned, but they don't exist today. The way that that would look is if you want to power a fully LLM-powered chatbot from another company it could be hooked into our platform with a third-party bot connector, and then the escalation to the agent happens the way it does with more traditional bot platforms.
More at scale, where you have customers who use Genesys alongside Dialogflow from Google Cloud – that’s been done for years and we’ll continue [supporting] that--there are other features like agent assist, where we do get that request. And that's the reason it's a roadmap item. [A customer may] have an investment and say hey, I've developed this model, I know it works,” [so, we’ll support that.]
NJ: So it seems Genesys has led with agent assist, but you don't necessarily need generative AI to provide similar assistance to agents. We’re assuming that there's a cost difference between those conventional AI tools, for lack of a better term, versus using the new generative models. So, how might a company approach that decision?
Weigl: I think there's sort of three tiers. There's more traditional AI methods, there's generative AI that is open source, and there’s “fit to use case” where we can run it on our own platform. We understand what the cost margin is because we've been doing it since 2020. Every time we get a request and need to provide a recommendation, we're actually hitting some other API. And that does get expensive.
However, I would also state that the economic characteristics of this ecosystem are going to get a lot more favorable pretty quickly. This stuff is going to be rapidly commoditized. A lot of people are doing a lot of really similar things, so it has a little bit of the curve of, you know, at one point, the VCR cost $10,000, and then, eventually it became something that’s a $50 item. Will that happen in a year? Probably not, but we do plan for a mix-and-match approach, where we know the highest-frequency elements won't get adopted by all of our customers unless it's cost favorable. I can't go out and charge $1,000 per agent per month for Agent Assist product, but I can charge about $25. So there's going to be a need to look at cost margin as an element of how you drive adoption of these features.
NJ: Do you see the more traditional predictive machine learning AI and generative AI coexisting?
Weigl: I think they are broadly compatible and there is a need for them to complement each other.
So if you take an example, the pure generative AI experiences are not particularly good at finding intent, because they'll start to make stuff up if they can't figure it out. You can do a little bit of input and output filtering, and tell the model don't make stuff up, but it's not a perfect science. But our predictive technology is specifically built to find intent and we have tools to figure out what customers want based on things they're typing.
So having that working alongside of and then training the generative AI, that's one of the biggest areas where we'll spend time and refinement, because we want to get to a point where we're confident that fully unsupervised self-service experiences can be fronted with a generative LLM. But you need the two sides working together.
There's a lot to suggest that, as well, from the ecosystem. OpenAI GPT 3 to GPT 3.5 to 4 – that leap in understanding was really aided and abetted by a lot of human feedback. Of course, that's not a new technique, I mean, where we started with this was everything had to be labeled [by humans] and that's how you trained [the models]. Now we went – because of compute and neural network advancements – to fully unsupervised training. Which is fine, but we're getting [better results] from layering human feedback on, as well, [which produces] even better refinement.
When we think about agent assist, we think about bots, but we really think about that as one conversational platform. One capability we're really excited about is agent-facing bots. This is on our near-term roadmap. As we open that up, it will have a human feedback loop that breeds more confidence in full self-service, because the agent will be able to observe what kind of answers that bot is giving.
NJ: What do you mean by an agent facing bot?
Weigl: You have a bot within the agent environment, so as the conversation advances instead of a widget that just gives [the agent] a suggestion based on what you think might be useful, like here's a knowledge article based on what the customer is telling you, it’s a more multifaceted bot that you can delegate work to and can ask when you have questions about the conversation that you're having, as well.
[With that agent-facing bot] we get the ability to maximize [their] workflow potential. [That agent won’t] have to “swivel chair” between systems or even deal with single-pane-of-glass issues in their environment. [And even in those solutions] you have a component for this system and a component for that system, and it might all look consistent, but [they] still require [the agent] to point and click through a series of tabs and figure out how to help the customer.
But an agent facing bot can flatten and front the access to all those systems and really simplify the delivery of service. And eventually, you know, that leads to fully self-service capabilities as well.
NJ: Where else do you see Gen AI being used in the contact center?
Weigl: I mean, broadly, we think of it as a series of assistive technologies. We're really interested in helping the agent, obviously, [since they’re] the most numerous user we have on our platform.
Helping the supervisor as well I think is huge, especially when you have a lot of supervisors sitting in front of dashboards, getting a lot of alerts. While they can dive into a few conversations and listen in real time and maybe take a conversation over, it [can be] a little hard to get a sense of what's going on. There's a lot to suggest that generative AI can present digests [to them and say] this is the one you should really worry about. [We think there’s value in providing] that kind of ambient assistance to somebody who's really busy and multitasking in the extreme.
We're also working on a lot of help for admins and content workers. So, for instance, generating a starting point knowledge base out of a bunch of content that they feed in. We already have some early versions of this that are non generative. You can point it at a URL and it will spider through, suck up all the content, build a draft and then index it for you so it's searchable. We already do that today. We're also working on connectors to some of the popular knowledge bases in the ecosystem. In addition to just supporting it natively [in our platform], generative AI can generate that content for you and then you're really editing and improving, rather than writing, which saves a lot of time.
And then, similarly, admins can benefit from things such as writing code for integrations based on a generative guidance principle rather than having to actually code it. Things like that, that really help with implementation or changes that need to happen in the environment are all items within our scope over the next couple of years.
NJ: Can you talk a little bit about what customers need to be aware of, maybe what types of questions they should be asking about data privacy, data security, etc.?
Weigl: If you buy Genesys Cloud, there's a lot of language in the master service agreement [MSA] that talks about this. And we have one of the most rigorous regulatory and compliance postures of any platform out there. We treat that pretty seriously.
The way that we talk about it with customers is on a refinement basis. That middle layer between the finished application and the foundational things that we do, or that we are based on, you have an immediate choice in the MSA about do you opt in or out.
The default is opt out. And a lot of the big ones, of course, just say thank you, you know, we don't want that. But, a lot of companies actually do [opt in] and they see the benefit of being able to get a better result that's tuned to them.
On the machine learning side and what we do with NLU has an anonymization pipeline that scrubs for any PII [personal identifiable information]. We operate in Europe and the teams building NLU are principally in Ireland. We will get into trouble if we don't do this right; we treat it seriously.
We have in that anonymization piece anything that anyone does with our out-of-box tools, like the intent finder that I mentioned, is that by definition that data is within your own organization only.
The additional nuance I would bring is just that, if we use a behind-the-scenes OEM, then we disclose that service. And then if you are contracting for something else and integrating it, that’s apparent to you because you've done it, right? And then you know, there is understanding on what data gets passed back and forth. [If there are] questions there, between those two vendors, [then] we get experts on the phone and make sure it’s [all] clear.
Want to know more?
Check out these articles and resources: